Alina Timmermann, M.Sc.
Phone and E-Mail
Tel.: (+49) 231 755 - 5427
E-Mail: alina.timmermann (at) cs.tu-dortmund.de
Office
Room 207
Otto-Hahn-Str. 16
Postal Address
Lehrstuhl Informatik 4
TU Dortmund
Otto-Hahn-Straße 16
44227 Dortmund
Deutschland
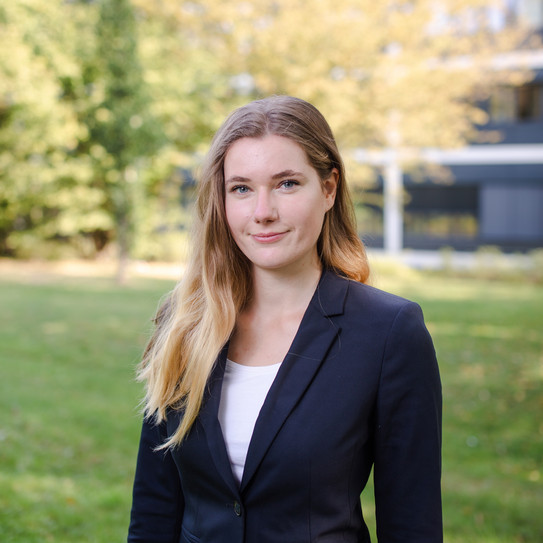
- Research Fellowship in Research Training Group 2193 "Adaptive Intelligence of Factories in Complex and Dynamic Environments".
- Sales forecasting for large industrial data sets (cooperation with Joyson Safety Systems)
- Clustering of time series, Ensemble learning for Forecasting
- Credible sales forecasting, trustworthy calculation of KIPs for forecast accuracy
- A. Timmermann, M. Abdulgader, L. Hagen, M. P. Milz, A. Wirtz, D. Biermann, W. Tillmann, F. Walther (2021), "Characterisation of arc-sprayed corrosion protection coatings post-treated by means of “machine hammer peening”", Thermal Spray Bulletin, Issue 01 (2021) Volume 14
- J. Baumann, A. Timmermann, D. Biermann (2021), "Generation of Predetermined Surface Structures by Simulation Based Process and Tool Design When Milling Free-Formed Surfaces", Sheet Bulk Metal Forming - TCRC73 2020. Lecture Notes in Production Engineering, Springer, DOI
- A. Timmermann, M. Abdulgader, L. Hagen, A. Koch, P. Wittke, D. Biermann, W. Tillmann, F. Walther (2020), "Effect of machine hammer peening on the surface integrity of a ZnAl-based corrosion protective coating", MATEC Web of Conferences 318 (4):01008, DOI
- D. Biermann, M. Tilger, A. Timmermann (2019), "Echtzeit-Aufnahme der Oberflächenrauheit im Rahmen einer zweistufigen Microfinishbearbeitung", Diamond Business, 70 (3), ISSN 1619-5558
01/2023
Nachfrageprognose mit Künstlichen Neuronalen Netzen bei limitierter Datenverfügbarkeit
08/2023
Klassifizierung von Kundenloyalität mifhilfe maschinellen Lernens
09/2023
Implementierung des Shifting-Bottleneck Verfahrens zum Scheduling in Job-Shops
- since 04/2022
Research Assistant and Doctoral Candidate, Research Training Group 2193, Chair IV: Modeling and Simulation, Faculty of Computer Science, TU Dortmund University - 01/2022 - 03/2022
Research Assistant, Chair IV: Modelling and Simulation, Faculty of Computer Science, TU Dortmund University - 01/2021 - 12/2021
Research Assistant, Chair VIII: Artificial Intelligence, Faculty of Computer Science, TU Dortmund University - 01/2020 - 12/2020
Research assistant and doctoral candidate, Institute of Machining Technology (ISF), Faculty of Mechanical Engineering, TU Dortmund University - 05/2016-09/2019
Master of Science, Industrial Engineering, TU Dortmund University - 2015
Technical internship at Captrain GmbH, Hecker Glastechnik GmbH, Microsonic GmbH (12 weeks) - 2013
Basic internship at Dr. Diestel GmbH (8 weeks) - 10/2012-04/2016
Bachelor of Science, Industrial Engineering, TU Dortmund University
- 2020
CES Sponsorship Award of the Association of German Engineers (VDI) for Master's Thesis - 2016 - 2019
Scholarship at Female Talents in Accounting and Finance program
- 2021-2022
Council member in the student dormitory Studentendorf: Organization of events for students - 2018
Schülerpaten e.V. Volunteer tutoring for students with a migration or refugee background - 2015
Mentoring Program for Erasmus Students at TU Dortmund University
Offered thesis topics (September 12th 2024)
Benchmark for the calculation and evaluation of forecast intervals
In addition to a point forecast, forecast intervals specify an interval in which the future value lies with a certain confidence (certainty). 95% forecast intervals are common, but quartile intervals and distributive intervals also exist, even if little research has been carried out. There are also numerous evaluation methods for prediction intervals, such as the coverage rate of the interval or the mean scaled interval score (MSIS), which penalizes intervals that are too wide. In this thesis, the aim is to bring together all these types and evaluation metrics of forecast intervals and compare their performance on a real time series data set.
Benchmark for residual analysis to evaluate the success of the forecast
There are various methods for assessing the success of a forecast, such as calculating the percentage deviation between the forecast and the actual value. As the actual value lies in the future in real cases and is therefore not available, the adjustment of the forecast model to the past values of the time series (fit) is considered instead. The difference, which is called the residual, is calculated from these two values. To evaluate the success of the forecast without future data, it could be tested, for example, whether the residuals are randomly distributed (random) and thus whether the forecast has recognized all patterns in the time series. In this thesis, all methods of residual analysis are to be collected and their performance validated on a real time series data set.
What is expected?
- Independent work
- High level of self-motivation, willingness and curiosity to familiarise yourself with a topic
- Basic programming knowledge
What is offered?
- Extensive support from the supervisor, in the form of 2 meetings per month and assistance with questions/problems
- Encouragement of the student's own ideas, creativity and skills